FinTech
FinTech
How FinTech and Machine Learning come together?
Financial world deals with immersive scale of statistical data and quantitative figures, which is a perfect field where machine learning triumphs.
Machine learning has widespread applications in various fields, such as medical science, hospitality, media and retailing. Similarly, financial industry is embracing the technology for its advancement in terms of rapid, sound and accurate solutions for data processing, analyses and inference.
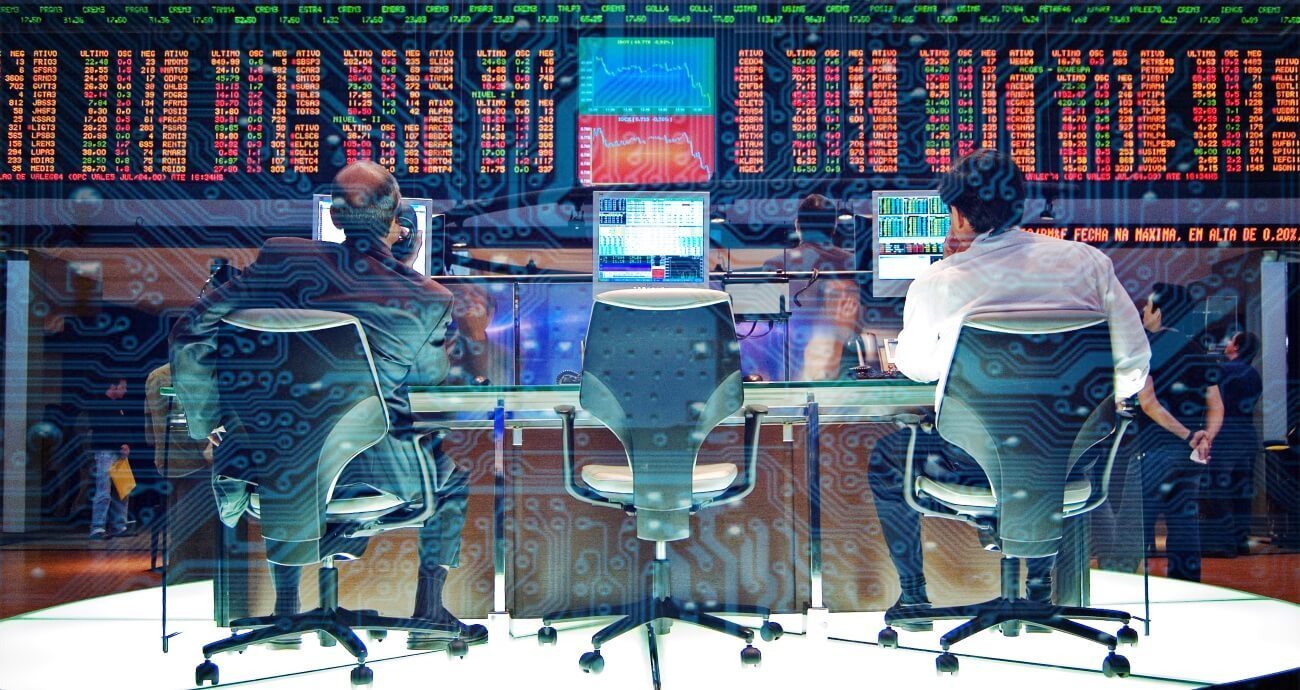
The financial world runs trillions of transactions every millisecond, commanding spontaneous response from every stakeholder. Machine learning dramatically leapfrogs the dimension of data management, boosting up response in speed of paramount importance.
Artificial Intelligence helps formulating unbiased financial decisions. Therefore, Machine learning algorithms can be trusted to unravel ill-conceived transactions, saving millions of losing trades.
Machine Learning in Finance
Most widely noted applications of machine learning are speech recognition, spam filtering, document processing, search engine analysis. In Fintech, machine learning is employed in Chatbot, Search engine, Analysis tools and multi-purpose mobile banking apps.
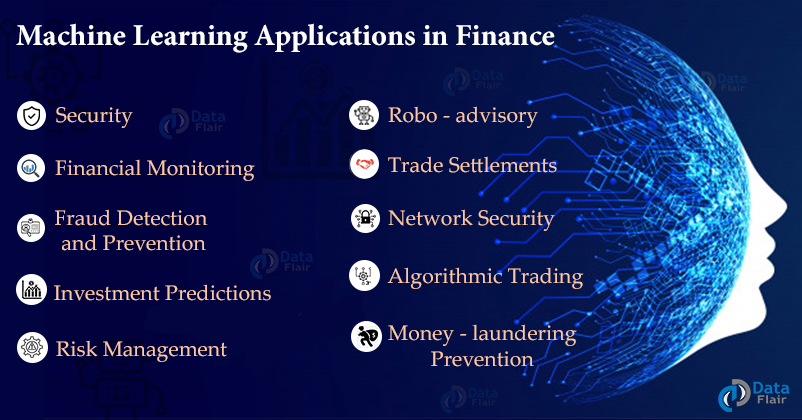
As mentioned, the financial ecosystem is a perfect field for artificial intelligence applications. Companies reportedly using machine learning are ever-increasing steadily, meaning it is an irreplaceable optimization more than a trendy gimmick.
Robo Advisor
The so-called “Robo Advisor” is playing up the role of financial expert, advising customers on financial status without undue human bias. It is an application where investment algorithms may be impartially adjusted according to client’s risk level and financial goals.

These performing algorithms include asset allocation, client risk analysis, portfolios adjustment or re-balancing, automated trading and taxation minimization. One of the observable advantage of Robo Advisor is the absence of sentiment in account management, therefore, this digital invention is relatively accessible than traditional financial advisory companies, making it attractive to a wider net of customer indifference to wealth level.
Equity trading firms can make use of over a few hundred of ML algorithms for various specific tasks such as trading prediction, identification of strategic parameters, market behavior analyses etc. For instance, building a strategic model requires decision tree algorithm, regression algorithms can solve regression problems and relationship of variables. ifundtrust is employing ML and AL for bond price valuation, risk assessment related analyses and other possibly imaginable.
Customer Service
Automated customer service to certain people has dismissive implication as many customers are unenthusiastic about following the voice prompt. But, customer service based on artificial intelligence promises different experience journey.

Dialog box and digital assistant will have attempt to respond and proactively route inquiries to the right responsible department. Further, its responses are human intelligible with adjustment to context, all without human intervention. AI recognize patterns and make use of context to comprehend and respond to special request.
Sentimental Analysis
This keyword literately means thorough analysis and identifying sentiment or opinion labels of given texts. The analysis has wide spread applications from establishing understanding of emotional reception on products and services to stock valuation and trade prediction.
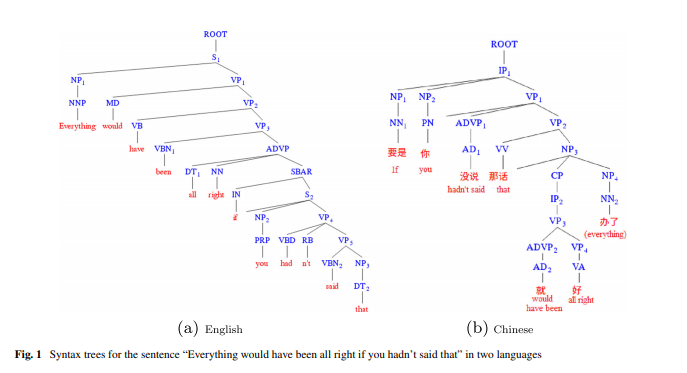
Recent advance in machine learning has substantially enhanced the quality and precision of sentimental analysis, even there remains visionary to show humane sixth sense (intuition) in machine. The potential remains astonishing with great potential.